The Problem
Model-based problem solving methods don’t work well for camera-based safety systems due to the complexity of modeling the large number of objects present on the road, such as vehicles, pedestrians, bicyclists, traffic signs, buildings, roadside objects and more.
The Question
What methods can we develop to train camera-based safety systems to recognize all objects present in the driving environment?
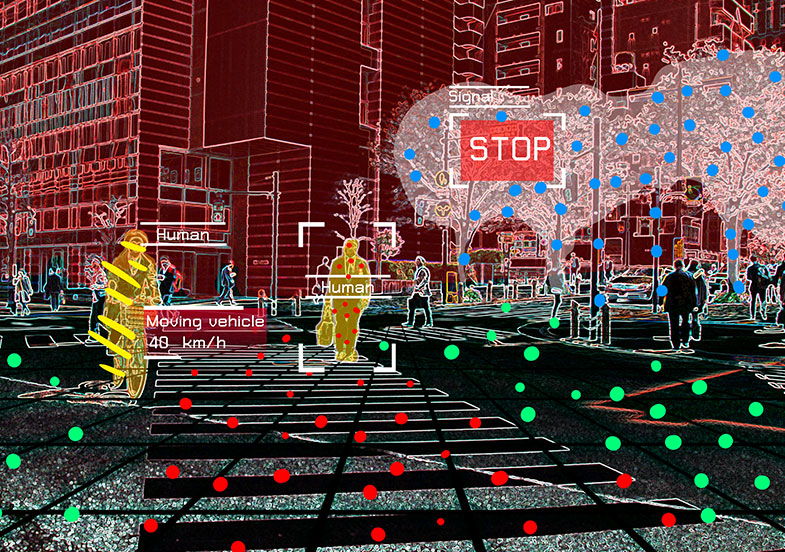
What We Did
With deep learning, automatic algorithms can be trained using a training dataset to better recognize a wide variety of objects in the driving environment. Utilizing these methods, we have been able to annotate over 1 million video frames with both fully automatic and semiautomatic methods.
The Result
As a result of this research, a deep convolutional network architecture was developed that can distinguish between drivable and non-drivable (unsafe) areas using camera imaging. 200,000 of these annotated frames will be made public through MIT to inform further research.
This is a project in collaboration with Massachusetts Institute of Technology (MIT) Age Lab